This page discusses statistical methods for disclosure control and how they impact common types of research output in Secure Transfer, Restricted-Use Data Lake (STRUDL).
Each section on this page is accompanied by a video explaining these concepts using the provided text as a visual aid. Documents referenced in each video are always formatted in bold and descriptions of each document can be found on STRUDL: Forms. We encourage potential applicants and program participants to reference the STRUDL Handbook for more details on the full program processes.
Video 5.1 defines Statistical Disclosure Control and explains some general practices researchers can apply to ensure their research products align with the standards applied during the STRUDL disclosure review process. Video 5.2 discusses some specific practices and methods for different types of output and the tradeoffs of their application.
Video 5.1: Defining Statistical Disclosure Control and General Practices for Research Output
Overview
In this section, potential applicants and program participants will learn about the following:
- the definition of Statistical Disclosure Control.
- general practices to align research products with disclosure review standards.
- general practices to align written products specifically with disclosure review standards.
- types of output that are comparatively safer from a privacy perspective.
What is Statistical Disclosure Control?
Statistical Disclosure Control (SDC), sometimes referred to as Statistical Disclosure Limitation (SDL), is a field of study. It aims to develop and apply statistical methods that enable the release of high-quality data products while reducing the risk of disclosure, or release of sensitive information contained in the data.
These methods have existed within statistics and the social sciences since the mid-20th century; researchers have likely encountered some of them before, even outside of the privacy context. SDC can be as simple as:
- suppressing (not releasing or withholding) certain records or results,
- aggregating variables into larger groups (like reporting state-level data rather than county-level data), or
- rounding numeric values to make them less distinct.
What are some general practices to align research products derived from confidential data with the disclosure review standards of Secure Transfer, Restricted-Use Data Lake (STRUDL)?
As depicted in Figure 1, here are some considerations for before, during, and after analysis that will make results more likely to receive approval or expedited review in the STRUDL disclosure review process.
- At all points throughout the process, remember that no information in any research product should identify an individual, household, or establishment;
- Before analysis, when possible, proposals should prioritize types of output with comparatively lower disclosure risk (discussed later on this page and depicted in Figure 2), including model or distributional output rather than tabular output;
- During analysis, results submitted for disclosure review must remain within the scope of the original STRUDL application;
- After analysis, remember that in addition to the review of tables, figures, and other output, publications and other written products derived from confidential data must also undergo disclosure review.
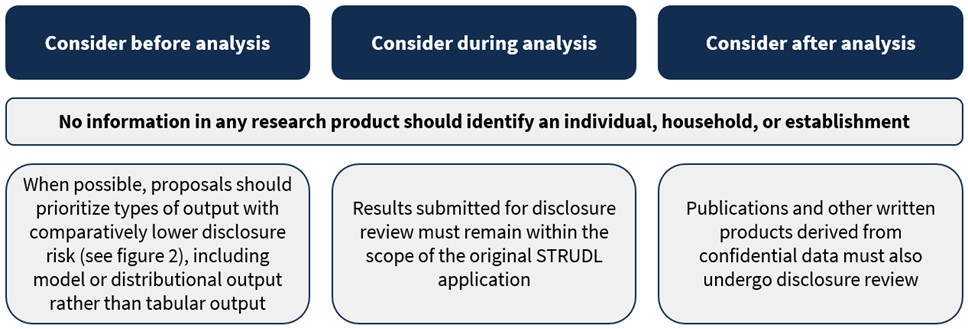
We will discuss specific methods that researchers can use later on this page in Video 5.2.
If tables and figures are already undergoing disclosure review, why must written products also be reviewed?
Publications and other written products derived from confidential data can pose a disclosure risk, as researchers may inadvertently reveal additional information about the data that a malicious actor can use to re-identify individuals, households, or establishments.
For example, consider the following illustrative sentences that could appear in a paper discussing results derived from restricted-use data:
Individuals were only included in our analysis if their income was below $30,000, the cutoff specified by the program. Only two individuals from the sample were excluded by this criterion.
While this information is not a “result” derived from the data, and thus unlikely to be included in a table or figure, it could still be used to re-identify individuals. In this case, despite not being part of the overall analysis, a savvy reader is now aware that the confidential data contained two individuals with an income higher than $30,000, and that their incomes are relatively anomalous in the sample. A malicious actor could use this information, along with other details about the data or cross-references with other available information, to re-identify one or both individuals.
Given this additional risk, all written products derived from confidential data must undergo disclosure review. The following practices will help align publications with RUD Access Program standards:
- all statistics referenced in the narrative must be included in tables and figures;
- no features of the data observed from the STRUDL environment should be discussed, unless this information has undergone disclosure review;
- no dates (including broad or coarsened information, such as seasons), geographical units, or unweighted sample sizes may be included without written permission; and
- inclusion and exclusion criteria for analyses of subpopulations should not be discussed in a manner that may inadvertently highlight small sample sizes, extreme cases, and unweighted counts that could be subtracted from totals.
What types of output pose less disclosure risk?
Some types of output are comparatively safer from a privacy perspective, and thus are more likely to receive approval or expedited review in the STRUDL disclosure review process. As depicted in Figure 2, the following types of output have comparatively less disclosure risk:
- Results derived from larger sample sizes;
- Results from large geographies; and
- Results for non-sensitive populations (examples of sensitive populations include individuals with veteran or disability status).
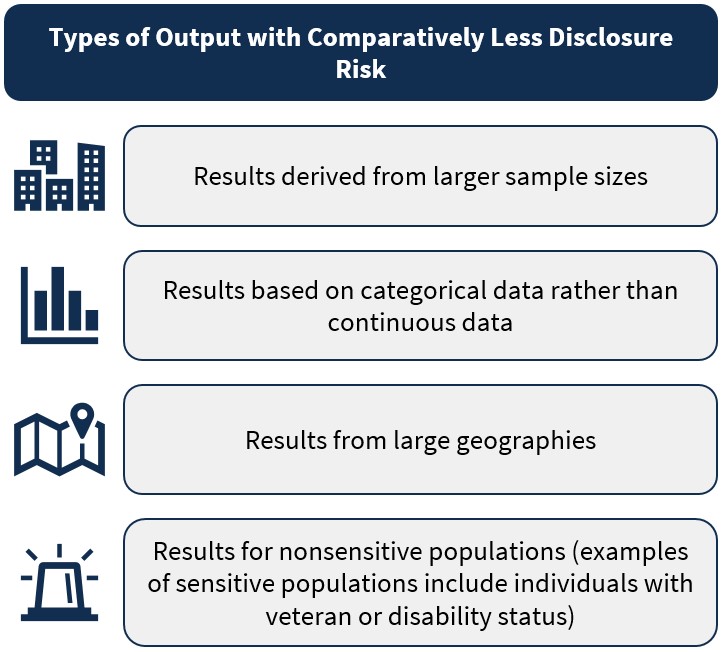
In Video 1.1, we discussed the privacy–utility tradeoff, the term privacy practitioners often use to refer to the tension between the overall usefulness of released data products and the privacy risk they pose. It is impossible to fully protect privacy or perfectly preserve utility.
The privacy–utility tradeoff may already be apparent from this list of comparatively safer outputs. For example, research about sensitive subpopulations can benefit equity and the overall public good. We share an application of this in Video 1.1, which discusses an evaluation of a DOL program providing employment services to veterans experiencing homelessness. However, these subpopulations are vulnerable to harms resulting from privacy violations, so this output must be reviewed with particular care to minimize risk.
Video 5.2: Applications of Statistical Disclosure Control Methods in the Secure Transfer, Restricted-Use Data Lake Context
Overview
In this section, potential applicants and program participants will learn about:
- traditional Statistical Disclosure Control (SDC) approaches and their applications to common analysis types; and
- modern SDC approaches that may be relevant to certain projects.
What are some traditional approaches to SDC?
Here we define some traditional approaches to SDC. As an illustrative example, consider Figure 3, an iconic painting by Georges Seurat, licensed under Creative Commons Zero, Public Domain Designation from the Art Institute of Chicago.
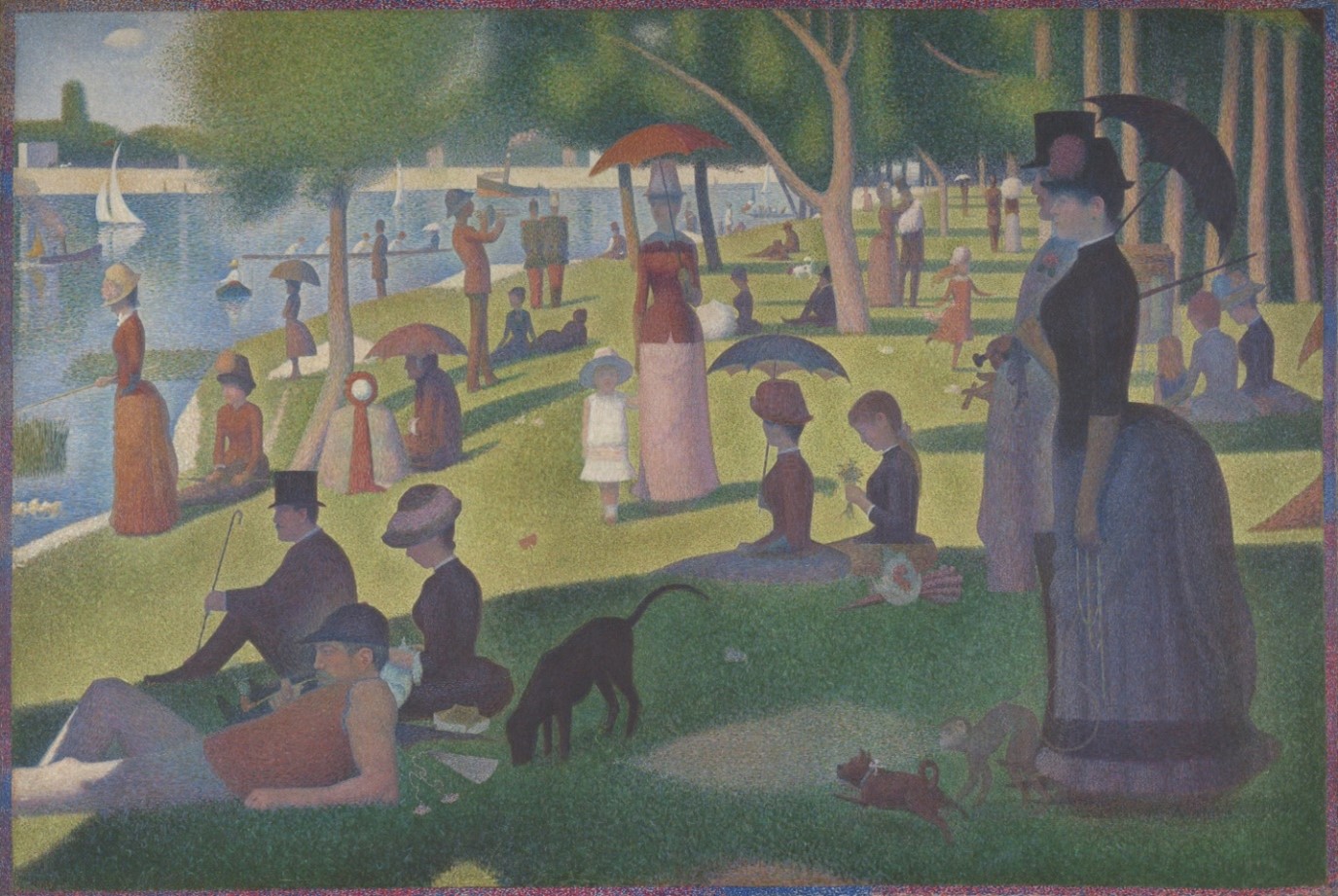
Each person in the painting in Figure 3 is currently re-identifiable. The goal of SDC methods is to preserve certain statistical qualities of the data (in this metaphor, the general image of the painting) while also maintaining privacy. We will demonstrate how each technique changes both the re-identifiability of the individuals and the larger image of the painting.
These illustrative images were originally published in Protecting Your Privacy in a Data-Driven World (CRC Press, 2021) by Dr. Claire McKay Bowen.
Suppression refers to omitting data or information about certain subgroups or data points (i.e., excluding counts or proportions that are based on very small sample sizes). In Figure 4, we can see that many individuals from the painting have been removed entirely.
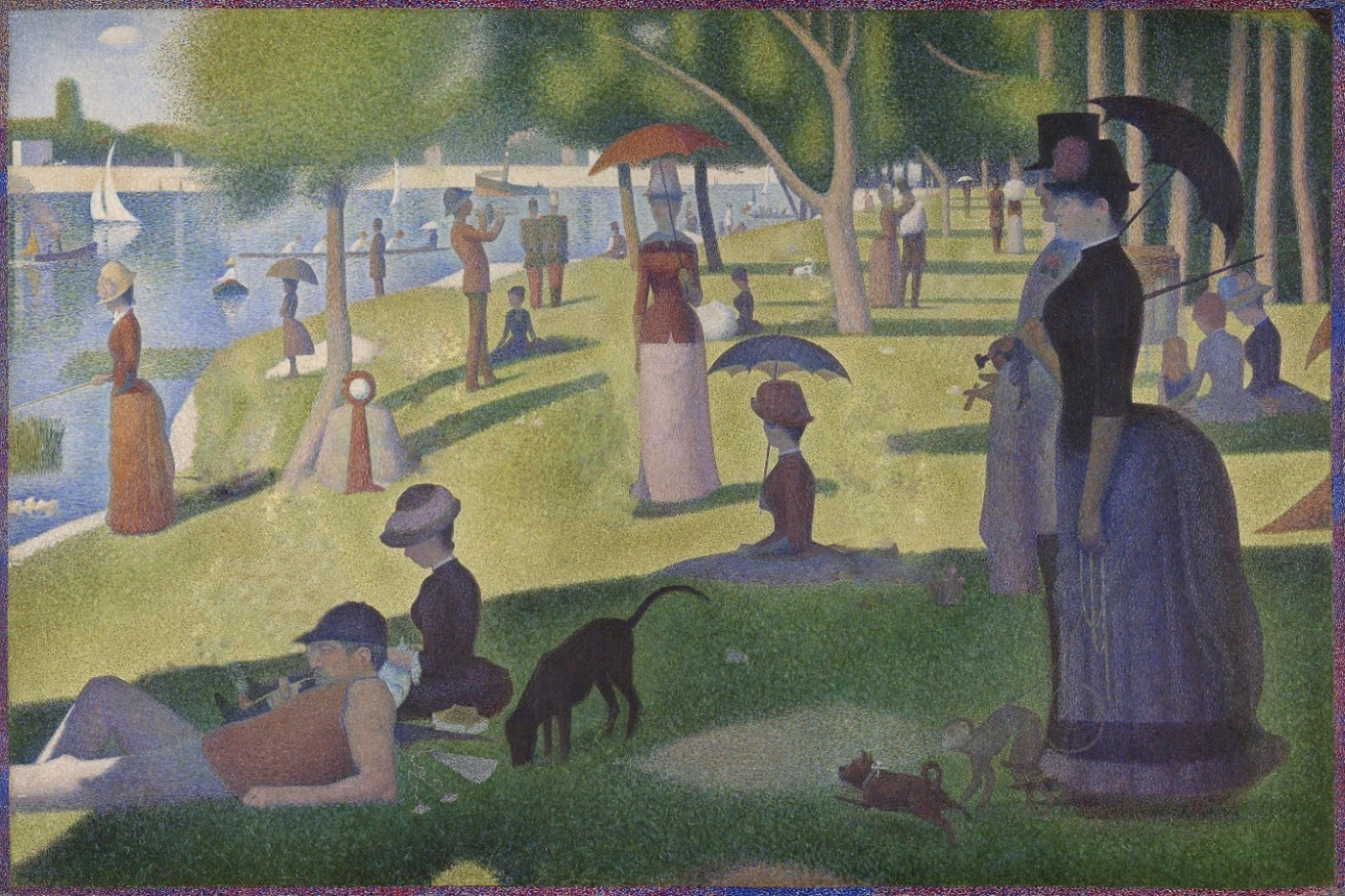
Generalization, sometimes called coarsening or aggregation, refers to combining more narrow levels of the data (i.e., counties) into broader levels (i.e., states). In Figure 5, the color palette of the painting is limited to a single shade of a given color, creating coarser groups.
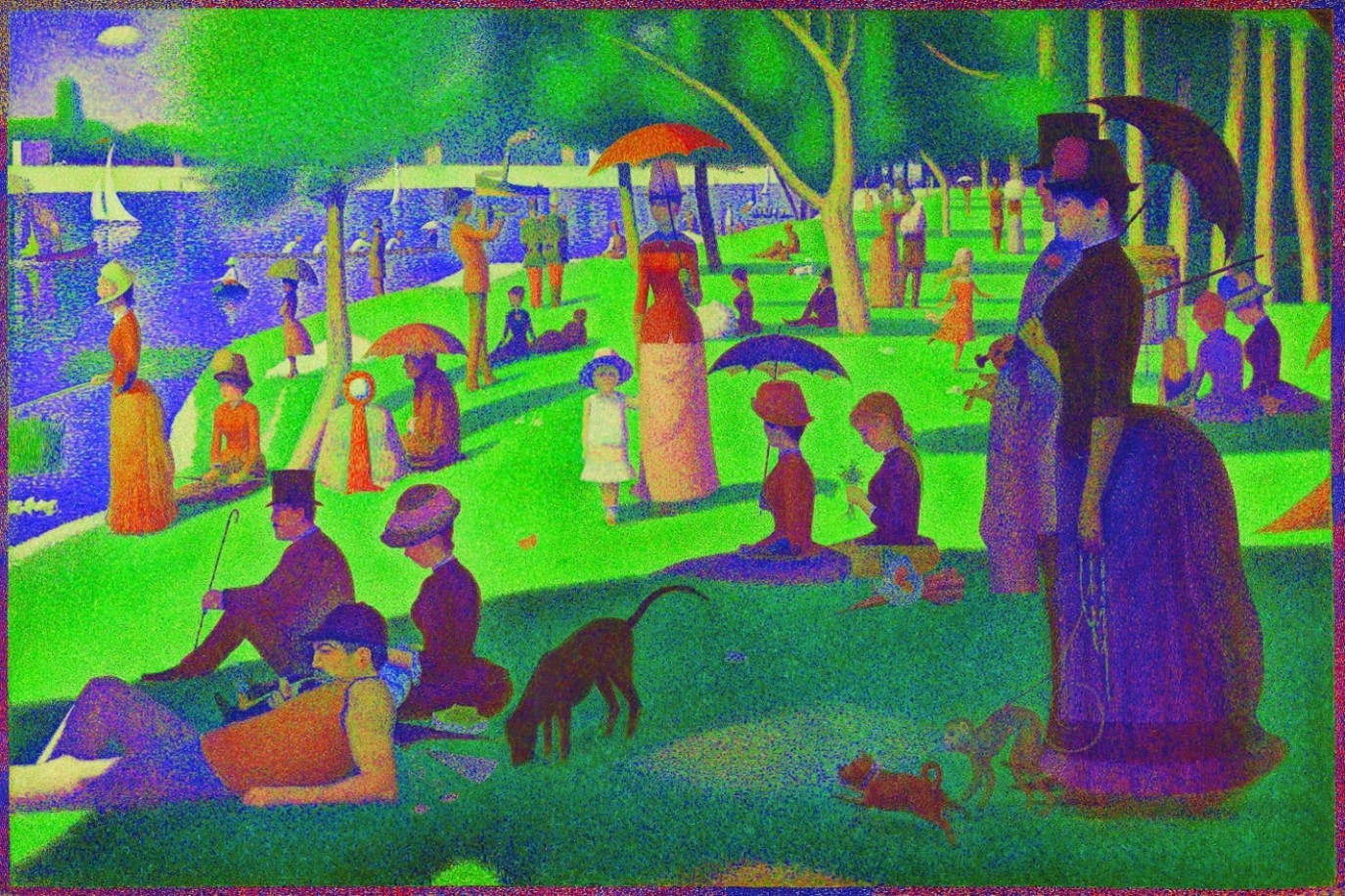
Noise infusion refers to adding random noise to continuous variables or numeric output. In Figure 6, pixels are lightened or darkened using random chance, making it harder to discern individual identities.
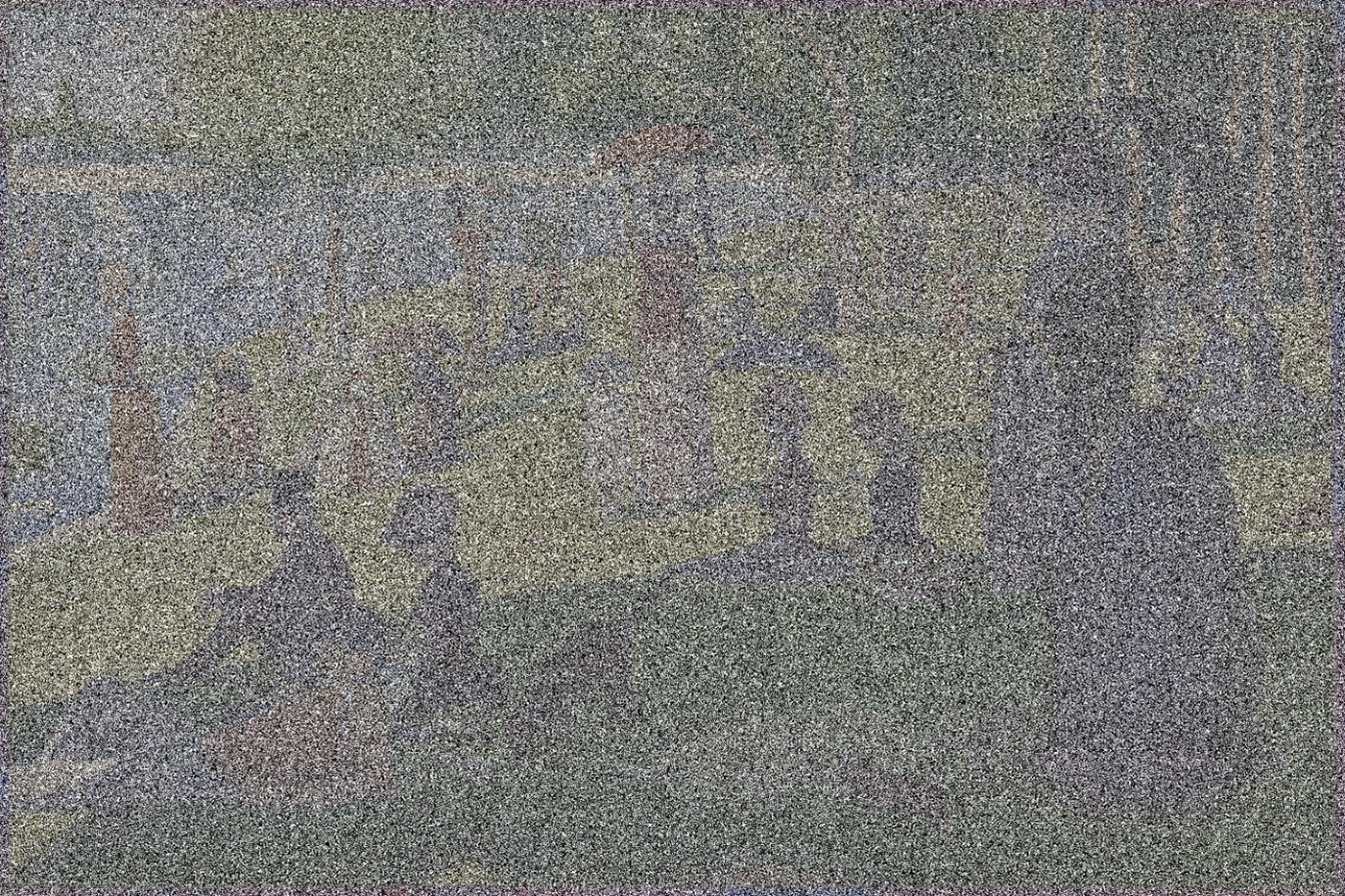
Top coding/bottom coding limits values above/below a threshold to the threshold value (i.e., individuals over age 95 are recoded to age 95 or a “95 and over” category). In Figure 7, we represent this process of recoding values above or below a threshold by changing the lighter colors to white.
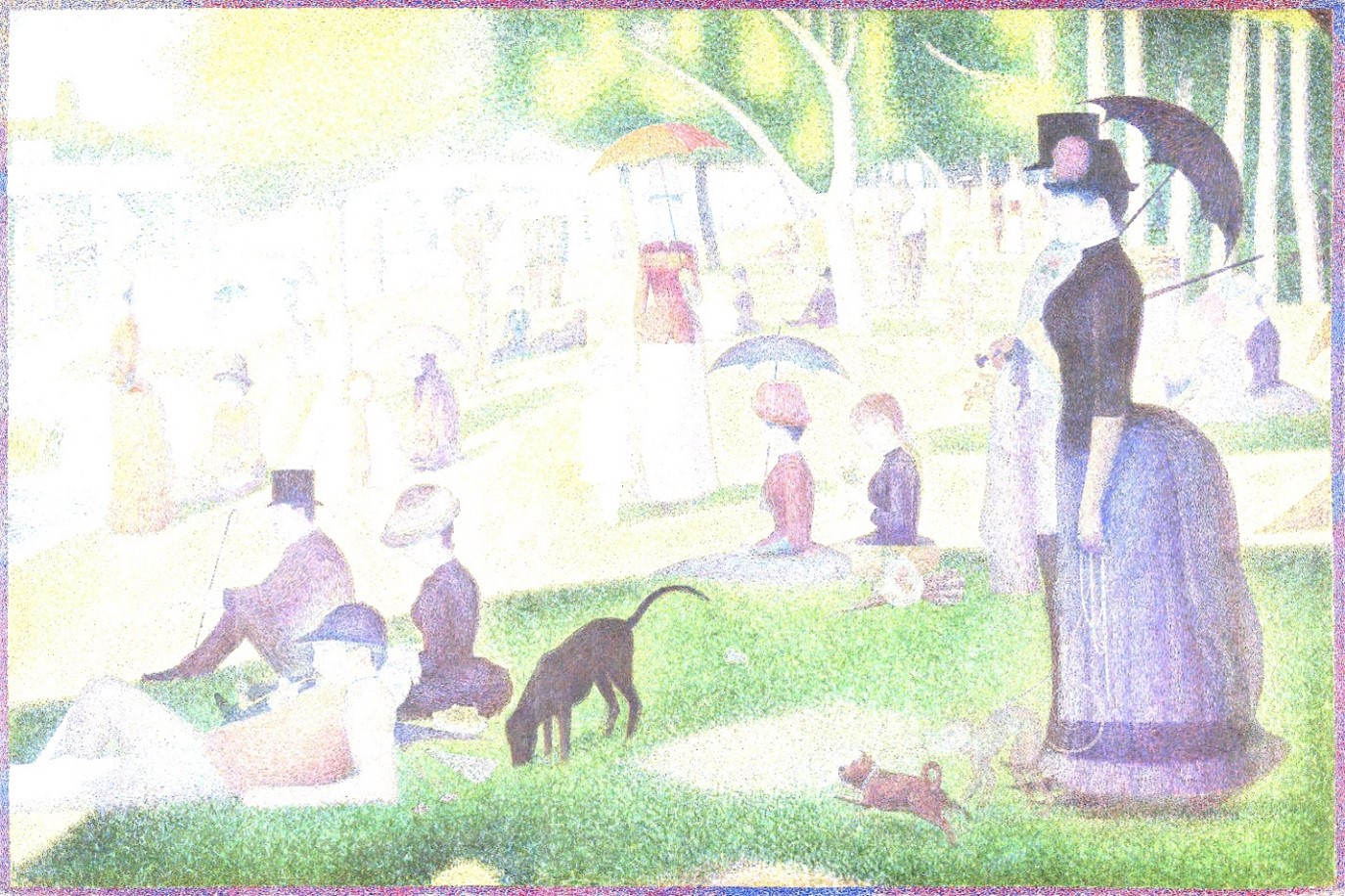
Rounding adjusts continuous variables or numeric outputs to be less exact. In Figure 8, lines around the objects in the painting are softened or blurred, making it harder to distinguish details.
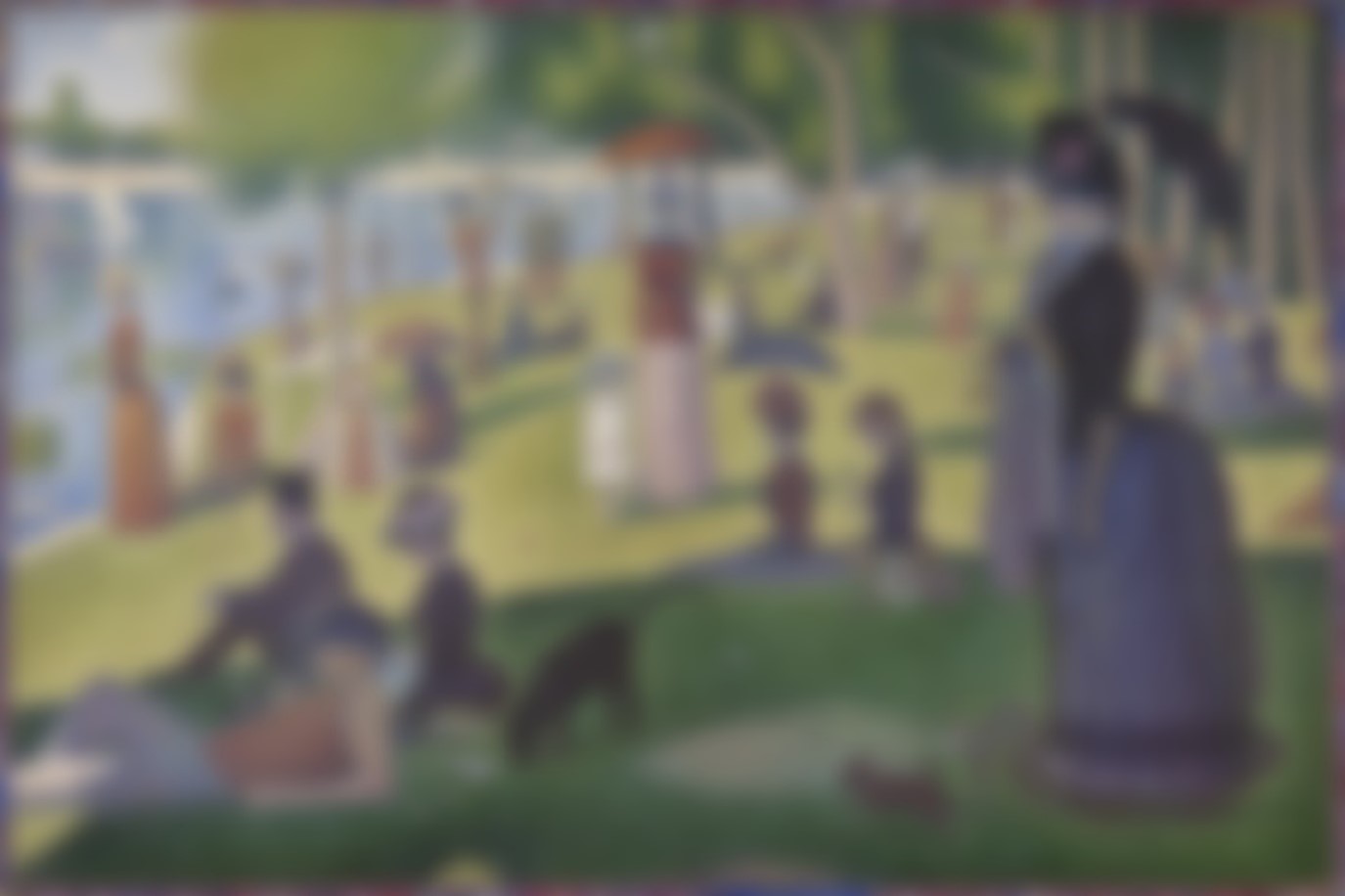
In addition to the methods depicted here, some other traditional SDC methods include, but are not limited to:
- sampling, or creating “plausible deniability” about whether a record was included by only releasing a sample of individual records; and
- swapping, or exchanging sensitive values among sample units with similar characteristics (i.e., exchanging income values between two households that are otherwise similar).
Which methods and standards will apply to a given research project?
It is difficult to be prescriptive about SDC, since each research project and its associated output pose different benefits (utility) and risks to privacy. The exact standards applied to a given research project will likely vary depending on the type of analysis, the sensitivity of the variables, and the output type (see Video 5.1 on this page for examples of comparatively safer outputs).
Approved researchers are encouraged to maintain an open dialogue with the STRUDL team about practices and methods that are best suited to their work and how to approach implementation: STRUDL@dol.gov.
What are some common applications and considerations when using these methods?
Approved researchers are encouraged to review the STRUDL Handbook for a more in-depth review of SDC methods as they may apply to STRUDL research output. The following table compares considerations and common applications for using these methods.
Approach | Considerations | Common Applications |
---|---|---|
Suppression | Suppression can affect marginalized subpopulations disproportionately, as it is often applied to subgroups, resulting in less representation in the data; this can create equity issues. Secondary, or covering suppressions, may be required to prevent inferences about the first round of suppressed values. | Statistics (i.e., counts, proportions, medians, minimum and maximum) based on fewer than k (a predefined threshold) observations may be suppressed. Data visualizations, such as scatterplots and point maps, may not be permitted (may be suppressed) if each point corresponds to a single record. |
Swapping | Swap rate (proportion of records with swapped values) cannot be shared publicly, or the swapping can be reverse engineered. | Swapping may be applied to sensitive variables in record-level data. |
Generalization (aggregation) | Tabular output that compares the exact number of records for combinations of more than two variables can be riskier than reporting marginal distributions of each two-way variable combination separately. | Generalization is often applied to aggregate record-level data to a higher unit of analysis. Generalization is often applied to geographic or temporal data. Generalization is often used in combination with suppression, where a count of individuals in a given category may be suppressed if it is smaller than a predefined threshold k. Generalization may also be applied to avoid suppression, if the broader categories allow for a larger sample size of individuals in a given category. |
Top coding/bottom coding | If top- or bottom-coding is applied to a numeric value (i.e., age 95) rather than a category (i.e., 95+), distributional output may be distorted. | Top- and bottom-coding are often applied to threshold values (i.e., maximum income to qualify for a program) or sensitive numeric variables (i.e., age). |
Rounding | Rounding rules can vary in complexity (i.e., probabilistic rounding determines whether a value is rounded up or down using a probability derived from the value). Different ranges of a numeric variable may apply different rounding rules (i.e., which significant figure is rounded). | Rounding is typically applied to sensitive numeric variables, or variables that make it easy to distinguish between observations (i.e., specific incomes). |
Adding noise | There are many ways to generate random noise, some of which can still preserve univariate distributions of the original variables. Once noise is added, postprocessing may be required to ensure values are still realistic in the context of the data. | Noise is often added to sensitive numeric variables or output. |
Sampling | Results based on sampled data should be weighted to account for the full data; reporting sample weights in this context may pose a disclosure risk. | Sampling is often applied to sensitive record-level data. |
What are some more advanced SDC approaches that may be relevant to STRUDL projects?
The list of methods discussed here is certainly not exhaustive. Some modern or state-of-the-art SDC methods, such as synthetic data or differential privacy, provide additional privacy protections and may apply to certain projects topics that would otherwise be too risky from a privacy standpoint. Potential applicants and approved researchers are encouraged to contact STRUDL @dol.gov during the application process to learn if these methods are a good fit for the prospective, or approved, project.